Latent variable models and deconvolution
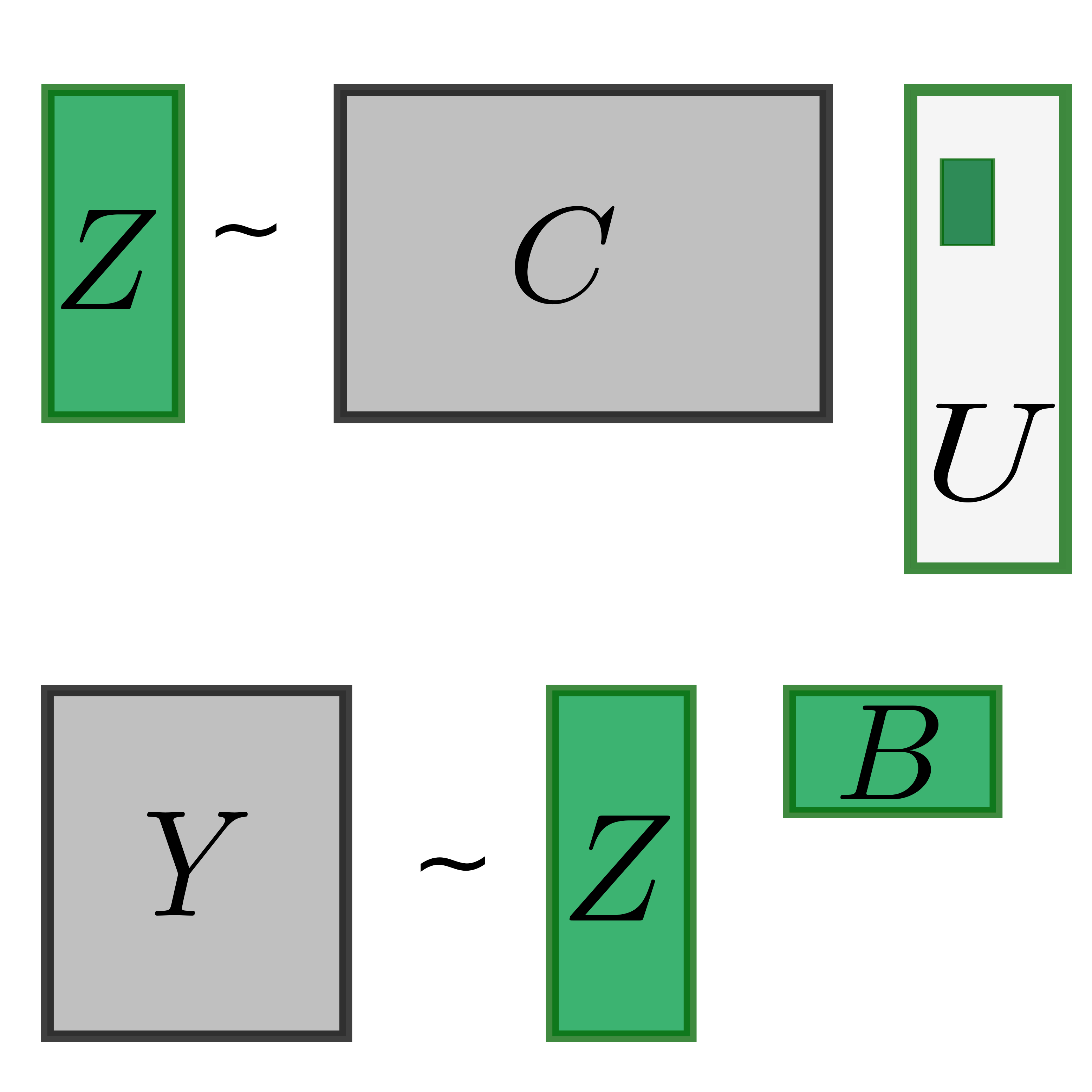
Functional genomic data (such as RNAseq or DNA methylation) are composed of many layers of overlapping signals that reflect the output of individual upstream pathways. We develop methods to automatically decouple, extract and name all biological latent variables present in a dataset.
- InstaPrism: an R package for fast implementation of BayesPrism - Bioinformatics (2024)
- Pathway-Level Information ExtractoR (PLIER) for gene expression data - Nature Methods (2019)
- Non-negative Independent Factor Analysis for single cell RNA-seq - Bioinformatics (2022)
- CellCODE: a robust latent variable approach to differential expression analysis for heterogeneous cell populations - Bioinformatics (2015)
Intrinsically interpretable models
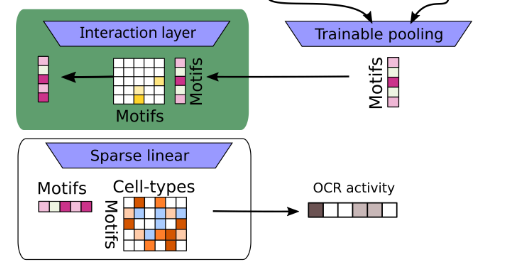
Advances in neural networks have enabled models that accurately recapitulate complex input-output behavior of biological systems. We can now predict context specific DNA activity and gene expression directly from sequence. However, top performing models have millions of parameters and their internal representation is not interpretable. We seek to develop models with interpretable parameters that do not sacrifice performance.
- An intrinsically interpretable neural network architecture for sequence to function learning - Bioinformatics (2023)
- Benchmarking of deep neural networks for predicting personal gene expression from DNA sequence highlights shortcomings - Nature Genetics (2023)
- Quick and effective approximation of in silico saturation mutagenesis experiments with first-order Taylor expansion - iScience (2024)
Convergent Evolution
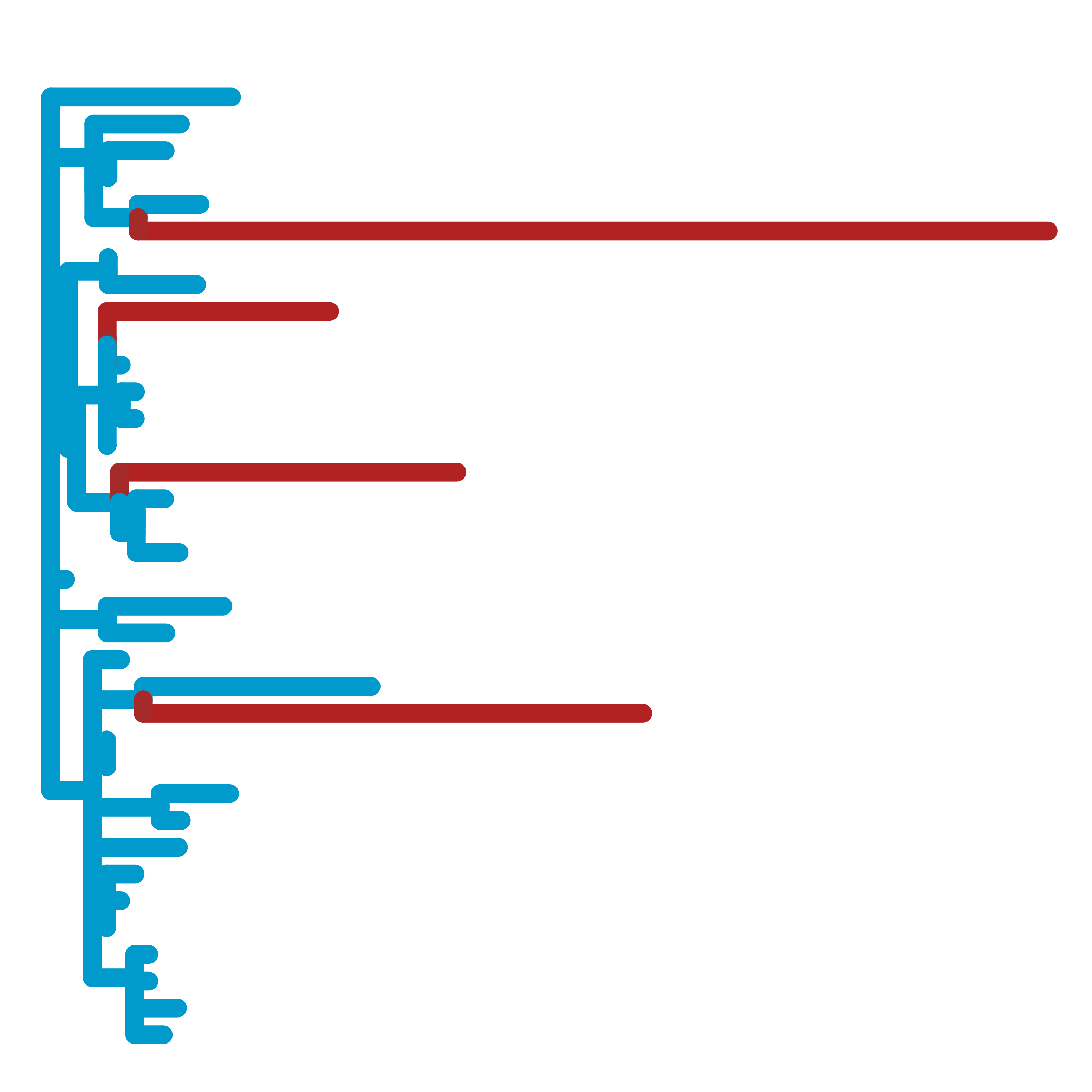
In collaboration with Nathan Clark's lab, we develop methods to uncover the relationships between evolutionary forces and phenotypes. Using our Relative Evolutionary Rate (RERconverge) method, we have identified genetic elements linked to subterranean and marine habitats, body mass and longevity, hair density, and more. This approach provides a powerful, complementary way to computationally map genotype-phenotype relationships.
- RERconverge: an R package for associating evolutionary rates with convergent traits - Bioinformatics (2019)
- Complementary evolution of coding and noncoding sequence underlies mammalian hairlessness - eLife (2022)
- Hundreds of Genes Experienced Convergent Shifts in Selective Pressure in Marine Mammals - Molecular Biology and Evolution (2016)
- Subterranean mammals show convergent regression in ocular genes and enhancers, along with adaptation to tunneling - eLife (2017)
- Pan-mammalian analysis of molecular constraints underlying extended lifespan eLife (2020)
- Ancient convergent losses of PON1 yield deleterious consequences for modern marine mammals Science (2018)
Automatic representation learning
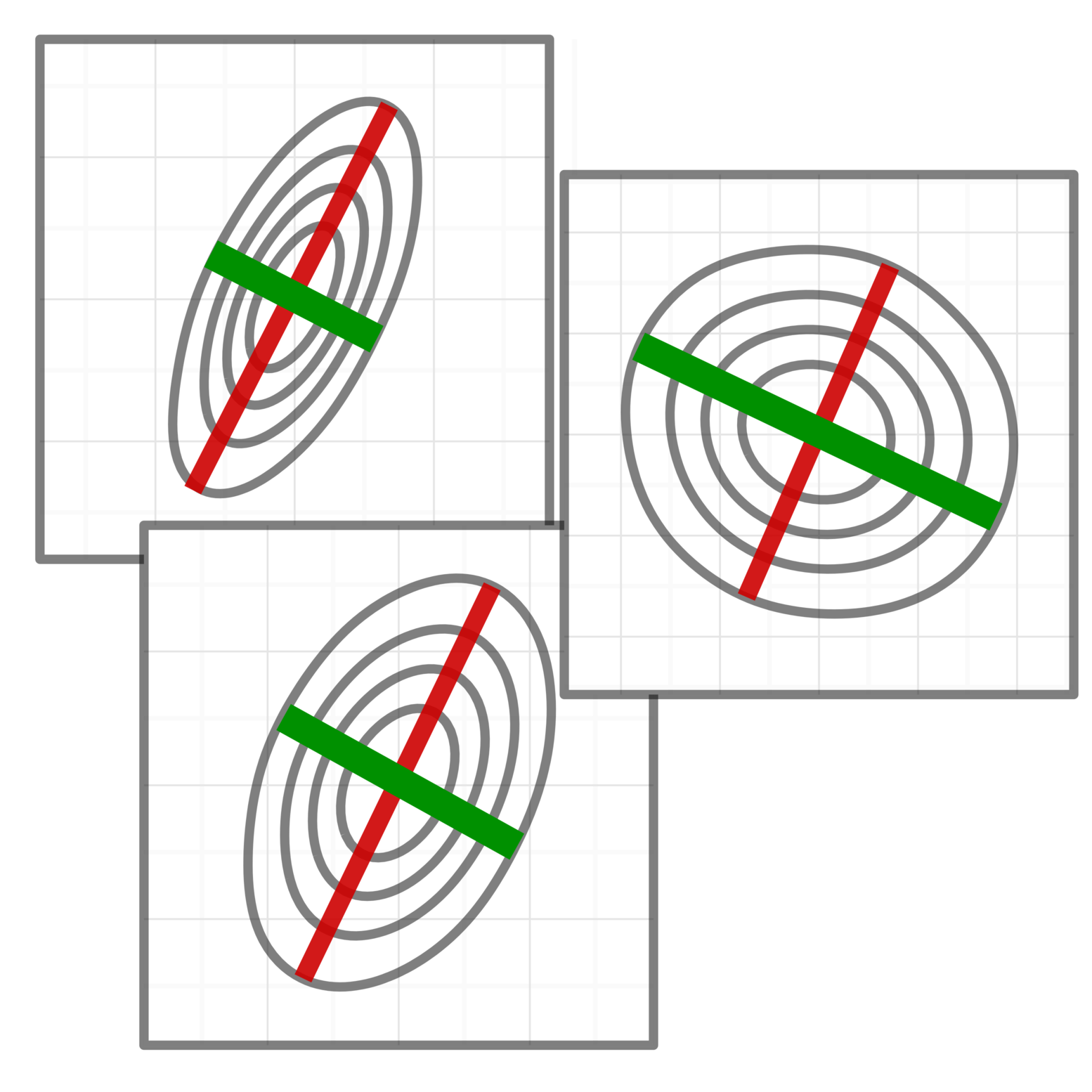
Genomic data is often noisy and complex making it difficult to identify signals relevant to the underlying molecular mechanisms. We develop methods that combine machine learning techniques and insights about the biological process to learn data representation tailored for specific downstream tasks.
- L0 segmentation enables data-driven concise representations of diverse epigenomic data - Bioinformatics Advances (in press)
- DataRemix: a universal data transformation for optimal inference from gene expression datasets - Bioinformatics (2020)
- Hybrid Bayesian-Rank Integration Approach Improves the Predictive Power of Genomic Dataset Aggregation - Bioinformatics (2015)
- A Hybrid Constrained Continuous Optimization Approach for Optimal Causal Discovery from Biological Data - Bioinformatics (2024)
Tumor immunology
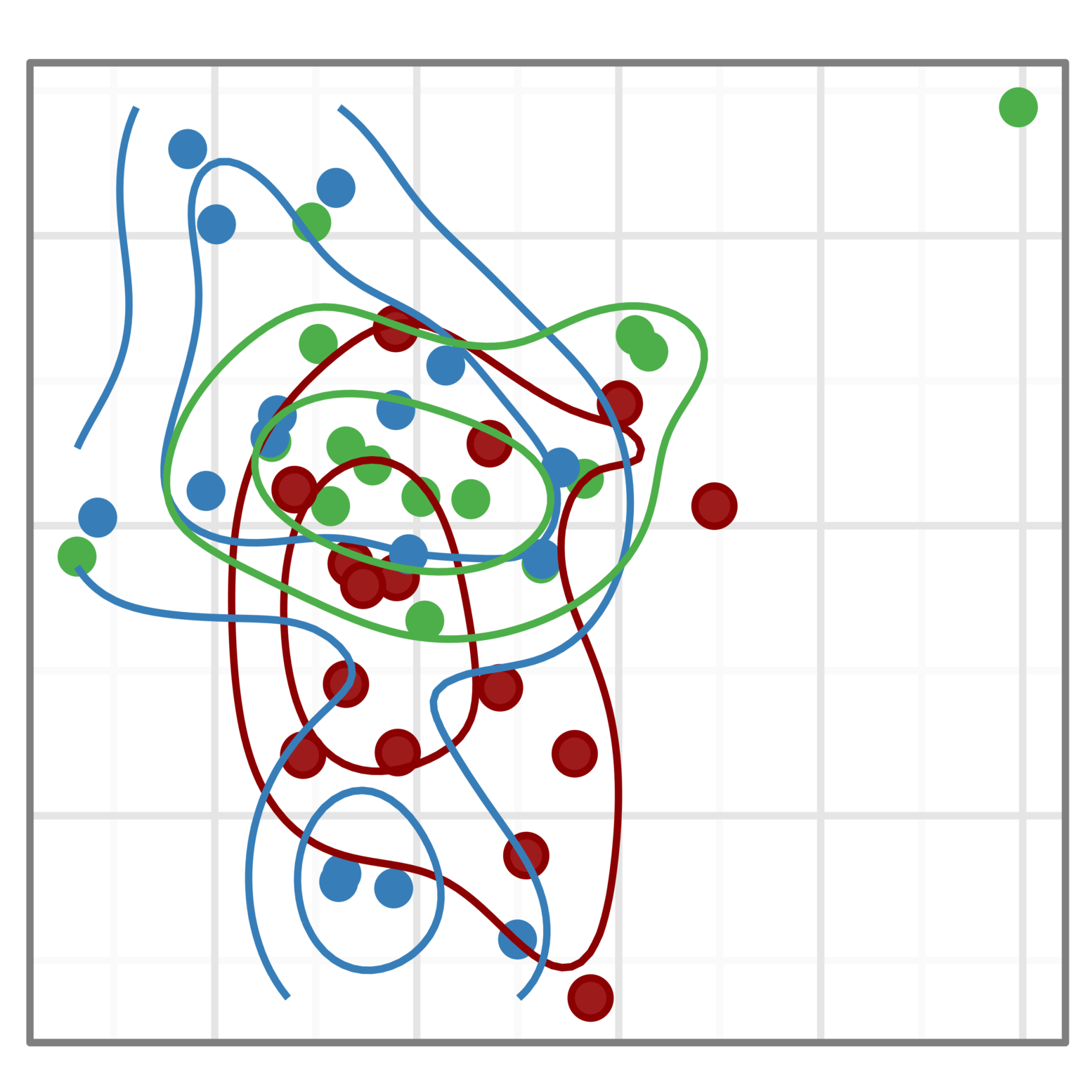
We are working with several UPMC research teams to use single-cell assay technologies to understand the role of the tumor micro-environment in tumor progression and treatment response.
- Heterogeneous pseudobulk simulation enables realistic benchmarking of cell-type deconvolution methods
- Regulatory T-cells promote the SREBP1-dependent metabolic fitness of M2-like intratumoral macrophages via CD8+ T cells - Immunity (2019)
- Interferon-γ Drives Treg Fragility to Promote Anti-tumor Immunity - Cell (2017)
Exercise Genomics
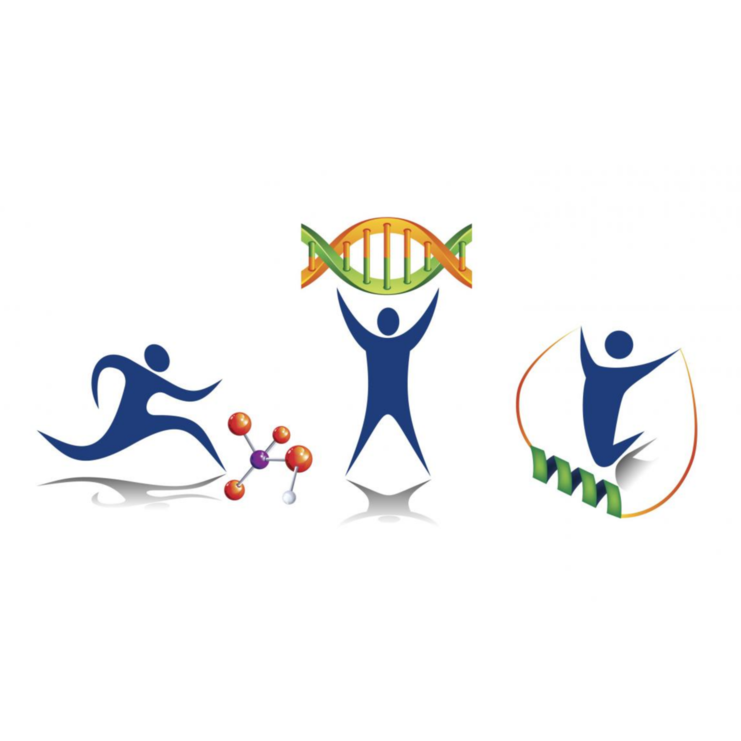
Our group is part of the Molecular Transducers of Physical Activity Consortium (MoTraPAC) . This is a large study looking at the effects of exercies through multiple genomic assays.
- Molecular adaptations in response to exercise training are associated with tissue-specific transcriptomic and epigenomic signatures - Cell Genomics (2023)
- Temporal dynamics of the multi-omic response to endurance exercise training - Nature (2024)
Epigenetic CHaracterization and Observation (ECHO)
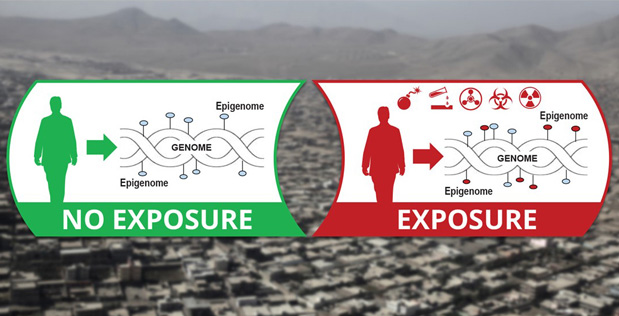
We are part of the Epigenetic CHaracterization and Observation (ECHO) . The program is building a man-portable device that analyzes an individual’s epigenetic “fingerprint” to potentially reveal a detailed history of that individual’s exposure to infectious and chemical agents.
- Human Dendritic Cell Response Signatures Distinguish 1918, Pandemic, and Seasonal H1N1 Influenza Viruses - J Virol (2015)
- Antibody responses to SARS-CoV-2 following an outbreak among marine recruits with asymptomatic or mild infection - Frontiers in Immunology
- A single intranasal dose of human parainfluenza virus type 3-vectored vaccine induces effective antibody and tissue-resident T cell response in the lungs and protects hamsters against SARS-CoV-2 - npj Vaccines (2022)
- Pre-infection antiviral innate immunity contributes to sex differences in SARS-CoV-2 infection - Cell Systems (2022)
- Earlier detection of SARS‐CoV‐2 infection by blood RNA signature microfluidics assay - Clinical and Translational Discovery (2022)
- A methylation clock model of mild SARS‐CoV‐2 infection provides insight into immune dysregulation - Molecular Systems Biology (2023)
- Multi-objective optimization identifies a specific and interpretable COVID-19 host response signature - Cell Systems (2022)